基于混沌壓縮感知和深度學(xué)習(xí)網(wǎng)絡(luò)的壓縮感知新模型
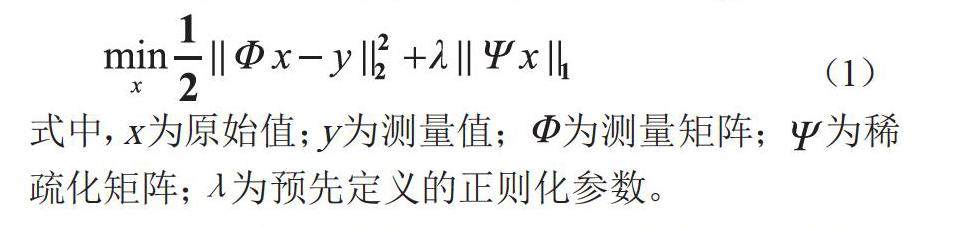
打開(kāi)文本圖片集
摘要:文章提出了一種基于混沌壓縮感知和深度學(xué)習(xí)網(wǎng)絡(luò)的壓縮感知新模型,稱(chēng)為混沌深度壓縮感知模型。該模型將傳統(tǒng)壓縮感知中的迭代步驟轉(zhuǎn)化為深度網(wǎng)絡(luò)形式,并將相關(guān)混沌參數(shù)應(yīng)用于測(cè)量矩陣生成和深度網(wǎng)絡(luò)訓(xùn)練過(guò)程。混沌深度網(wǎng)絡(luò)中的所有參數(shù)都將通過(guò)程序自動(dòng)學(xué)習(xí)獲取,不再需要人工設(shè)計(jì)。
關(guān)鍵詞:壓縮感知;深度學(xué)習(xí);神經(jīng)網(wǎng)絡(luò);混沌理論
doi:10.3969/J.ISSN.1672-7274.2023.02.032
中圖分類(lèi)號(hào):TP 391.41 文獻(xiàn)標(biāo)示碼:A 文章編碼:1672-7274(2023)02-00-03
A New Compressed Sensing Model Based on Chaotic Compressed Sensing and Deep Learning Network
CHEN Yixin, MA Zeng
(Basic Department of Naval Submarine Academy, Qingdao 266000, China)
Abstract: A new compressed sensing model based on chaotic compressed sensing and deep learning network is proposed, which is called chaotic deep compressed sensing. The iterative steps in traditional compressed sensing are transformed into deep network form, and the relevant chaotic parameters are applied to the measurement matrix generation and deep network training process. All parameters in the chaotic depth network will be acquired automatically through program learning, and no manual design is required.
Key words: compressed sensing; deep learning; neural network; chaos theory
0 引言
壓縮感知理論證明,當(dāng)一個(gè)信號(hào)在某些變換域表現(xiàn)出稀疏性時(shí),它能夠以較高的概率使用比奈奎斯特抽樣理論所確定的少得多的測(cè)量值重構(gòu)[1]。(剩余3722字)