基于KGCN與RippleNet的推薦系統(tǒng)核心特征提取研究
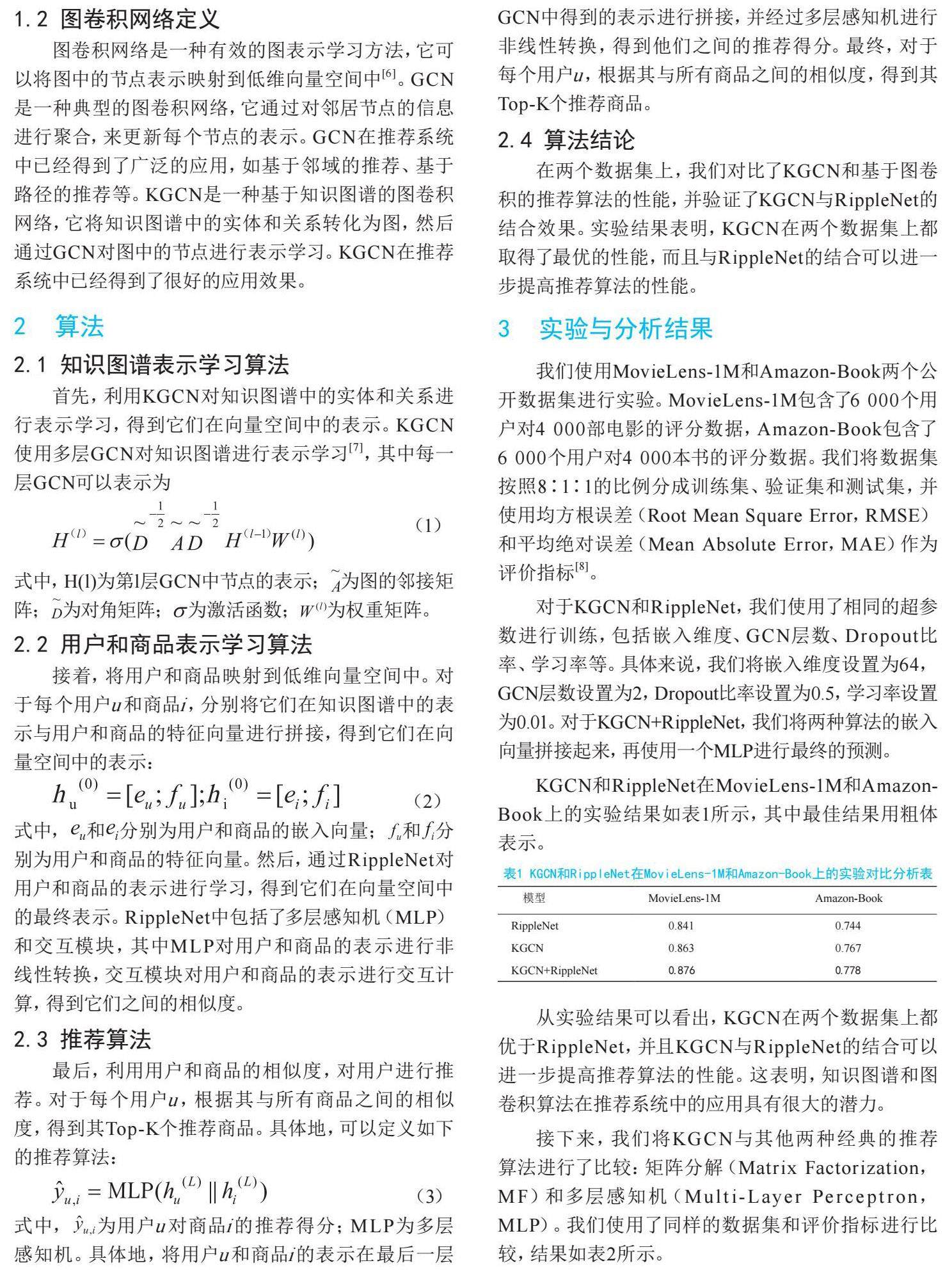
打開文本圖片集
摘要:文章提出了一種將KGCN和RippleNet相結(jié)合的方法,其利用KGCN提取知識圖譜中的實(shí)體和關(guān)系信息[1],利用RippleNet對用戶和商品進(jìn)行表示,從而提高推薦效果。實(shí)驗(yàn)結(jié)果表明,該方法可以顯著提高推薦效果,并提取出一些核心特征。
關(guān)鍵詞:推薦系統(tǒng);知識圖譜;圖卷積網(wǎng)絡(luò);KGCN;RippleNet
doi:10.3969/J.ISSN.1672-7274.2023.06.010
中圖分類號:TP 183,TP 391.1 文獻(xiàn)標(biāo)志碼:A 文章編碼:1672-7274(2023)06-00-03
Abstract: The article proposes a method that combines KGCN and RippleNet, which utilizes KGCN to extract entity and relationship information from the knowledge graph [1], and uses RippleNet to represent users and products, thereby improving recommendation effectiveness. The experimental results show that this method can significantly improve recommendation performance and extract some core features.
Key words: recommendation system; knowledge map; graph convolution network; KGCN; RippleNet
推薦系統(tǒng)利用電子商務(wù)網(wǎng)站向客戶提供商品信息和建議,幫助用戶決定應(yīng)該購買什么產(chǎn)品,模擬銷售人員幫助客戶完成購買過程[2],已經(jīng)被廣泛應(yīng)用于電商、社交媒體等領(lǐng)域。(剩余4265字)